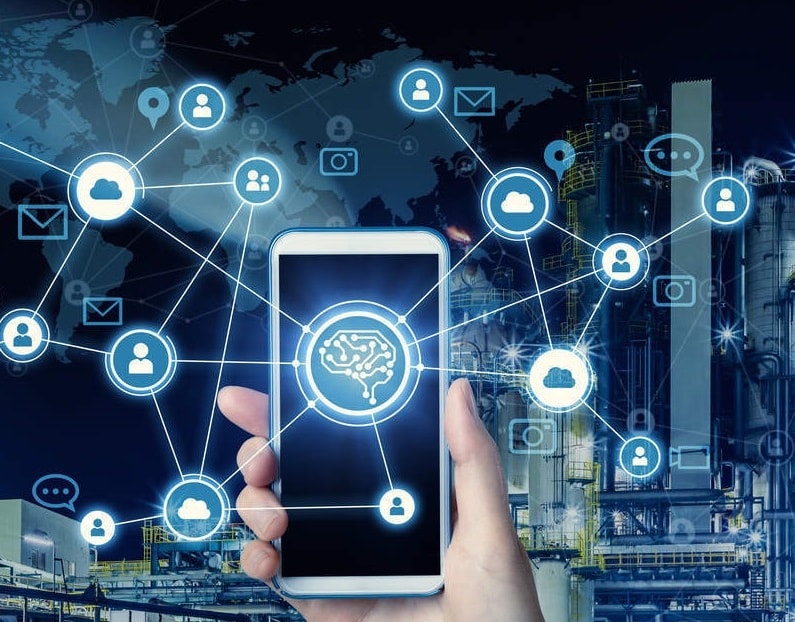
Using AI -- Avoiding Financial Losses and Making Financial Gains
The ability to predict in a market economy is equal to being able to generate wealth by avoiding financial losses and making financial gains. It would offer a significant and lucrative competitive edge over other market participants.
Behavioral economics tells us that emotions can profoundly affect individual behavior and decision-making. Sentiment analysis is an area where structured and unstructured data is analyzed to generate useful behavioral insights leading to improved performance.
Nowadays, many investment banks and hedge funds are trying to utilize the sentiments of investors to help make better predictions about the financial market.The challenge is to transform [market] information into an increase in the value of their asset holdings, that is, capture the ever-elusive alpha. Where and how can the firms innovate to obtain such alpha?