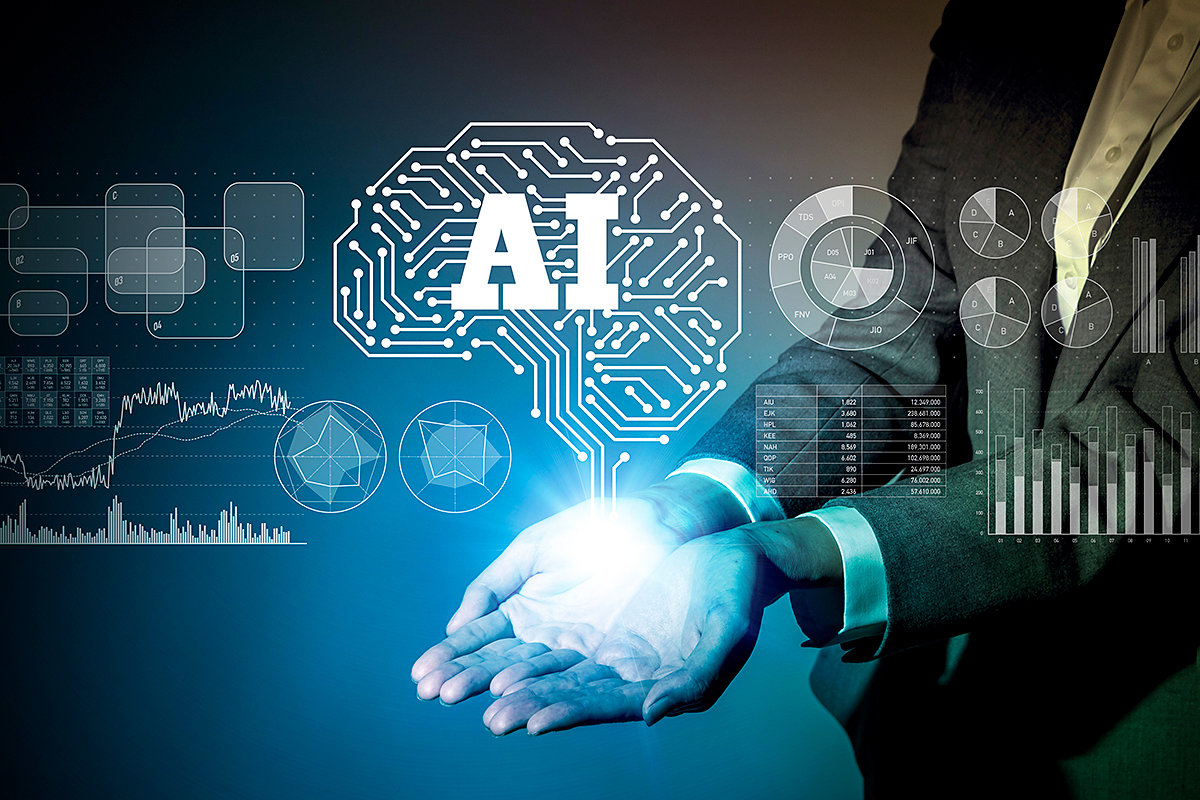
Credit Risk Analytics
At GPS.ai, we are using machine learning and artificial intelligence to transform the banking industry, using vast amounts of data to build models that improve decision making, tailor services, and improve risk management.
We help clients optimize their risk exposures, improve performance, increase profits, and accelerate growth.
Our advanced credit risk analytics enable institutions to improve underwriting decisions and increase revenues while reducing risk costs. We work across all asset classes, credit risk models, and the entire credit life cycle, including profit maximization, portfolio management, and loss mitigation.